During the image capture process, there are various parameters that define the final result obtained, such as, exposure time, sensor ISO, chosen aperture, or lens focal length. Among these, the ones that are most relevant for nighttime photography are, without a doubt, exposure time, sensor ISO, and lens aperture.
The ISO of a lens was named by the International Organization for Standardization to refer to the sensitivity of the film in a camera to light. Although most current cameras use electronic sensors and film has been relegated to more artistic fields, it is still a measure used for these sensors today. The higher the ISO value of a sensor, the more light is captured, resulting in an image with brighter tones.
On the other hand, the aperture, as its name suggests, limits the amount of light entering the lens system by placing an obstacle. As we increase this obstacle, the amount of light reaching the sensor decreases, resulting in less bright images.
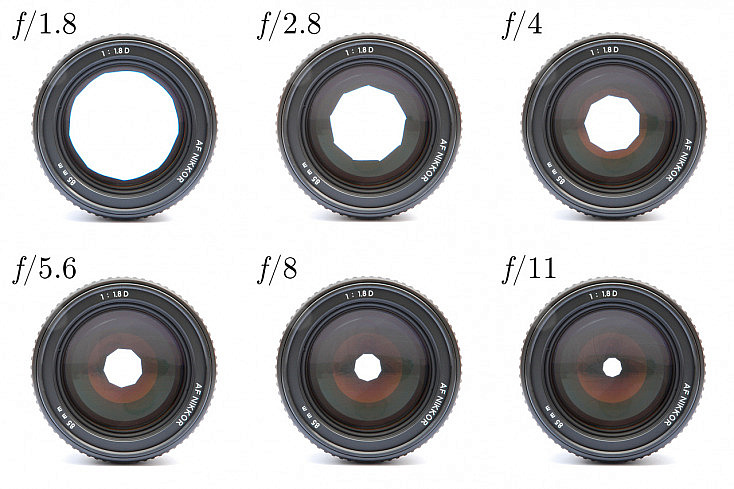
Lastly, exposure time (also known as shutter speed) represents the total time the sensor is exposed to the scene it is trying to capture. With a longer exposure time, brighter images are obtained due to the greater total amount of light that the sensor receives.
Based on what has been said so far, it seems that to capture images, we should maximize these three parameters. However, the first problem we might encounter becomes more severe by doing this: image oversaturation. Since camera sensors have a limited range of intensities (8, 16, 20-bit sensors), excessively increasing these three parameters causes the sensor’s pixels to register values close to the maximum, creating a glare effect. Due to this first problem, these three parameters are calibrated with each other to obtain the best image. When capturing images in low-light scenes, aside from this problem related to the camera’s characteristics, we may encounter two other significant issues.
In low-light situations, the noise generated in an image is amplified. There are various sources of noise in an image, but generally, this can be approximated by the addition of two different types of noise: Gaussian noise and shot noise. The first is noise inherent to the physical capture process, dependent on the lighting and the device’s temperature. On the other hand, shot noise is caused by the quantum fluctuations of photons, which lead to intensity variations in neighboring pixels. In low-light conditions, this second type of noise becomes significant because the intensity variations of the pixels become more noticeable than in well-lit conditions, and it follows a Poisson distribution.
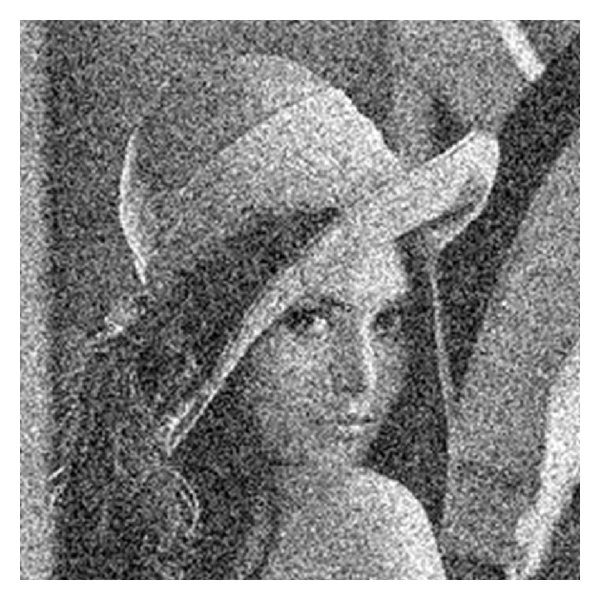
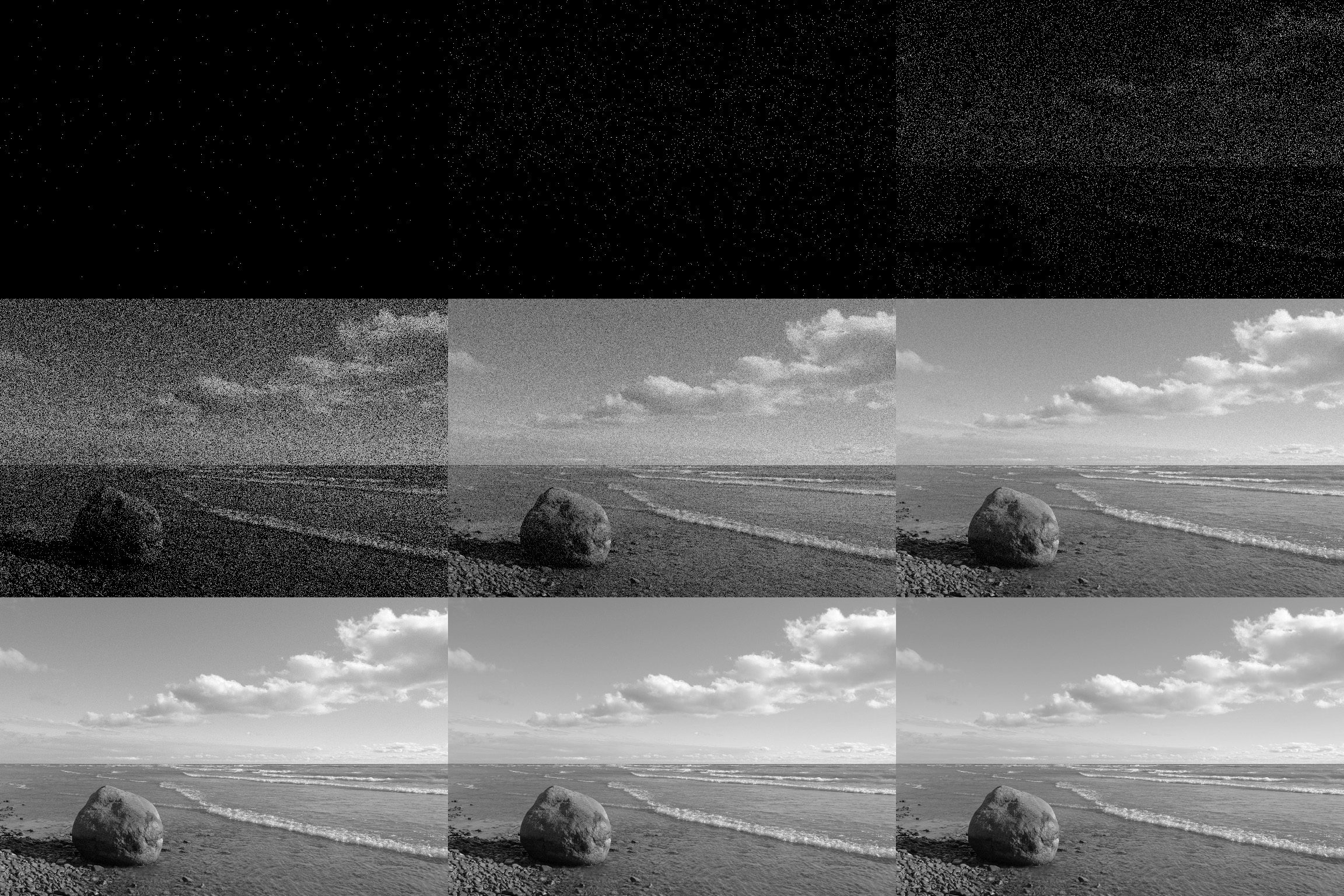
As we see in the figures, this noise can be visually catastrophic and needs to be reduced. In the case of shot noise, this can be achieved by increasing the amount of light that the sensor receives. When increasing the aperture or ISO does not improve the visual result of the image, it is necessary to increase the exposure time. It is then that the last problem I want to discuss arises.
During the capture process, it is ideal that the scene remains unchanged. For example, when we try to capture a moving car, it usually appears blurry in the final result. Some cameras manage to capture moving objects with good quality, but they require very low exposure times and large apertures. Equipment that meets these two requirements tends to be more expensive, making it not a viable option for many applications. In addition to the movement of external objects, there is also the involuntary movement of the hand of the person taking the picture. This can again be corrected by using tripods or stabilizers, but this is not always feasible in every case. Exposure time plays a significant role in these blurring effects, as reducing exposure time decreases blurring.
Thus, we see how this triangle of parameters that provides a better image involves many nuances, and the decision on parameters is made considering the requirements. Therefore, as we have concluded, when the scene has low light, both noise and image blurring increase. If this cannot be corrected at the time, either due to poor camera settings or limitations of the camera, the quality can be improved through image post-processing.
Currently, the most advanced image post-processing techniques include the use of deep learning. For low-light scenes, there is a wide range of work that significantly improves images compared to methods from 20 years ago. There are also many methods for handling blurry images, making it a continuously evolving field. However, as previously discussed, low-light images often have more blurring, and there are few studies on these situations. Therefore, we have worked to propose a solution for these two types of degradation. For interested readers, the article can be consulted in [ADD CITATION]. In the next Figures there are examples of the capabilities of this model.
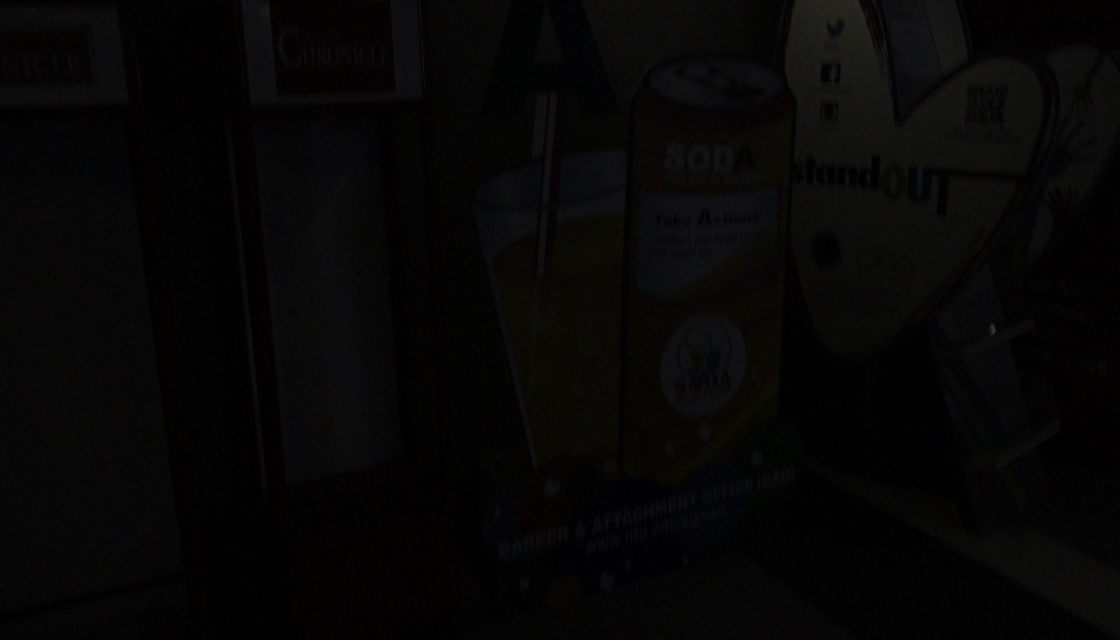
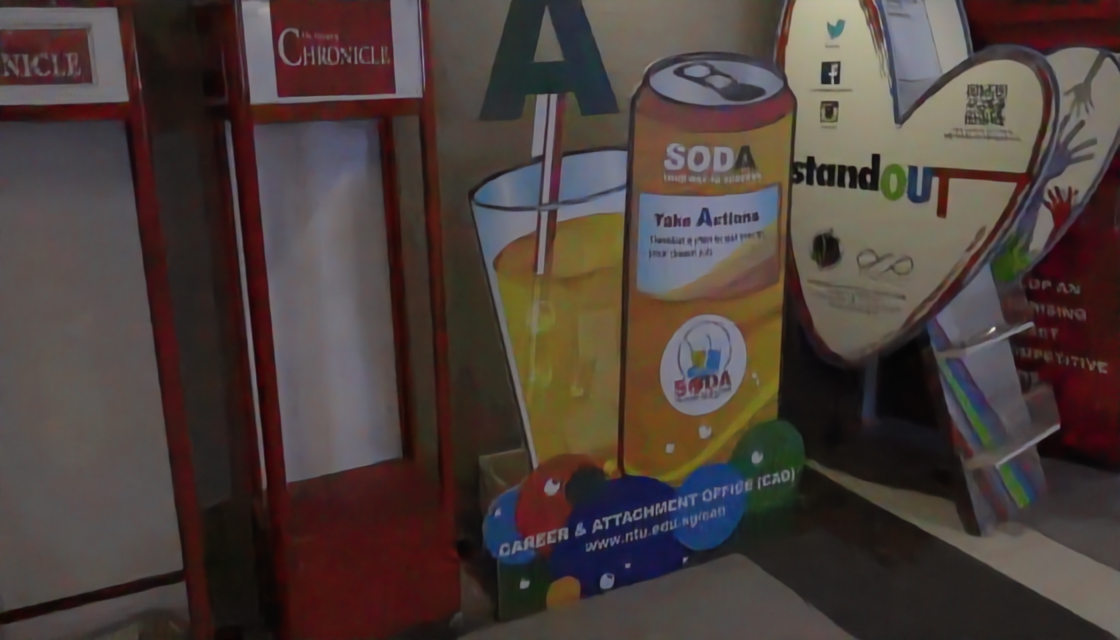
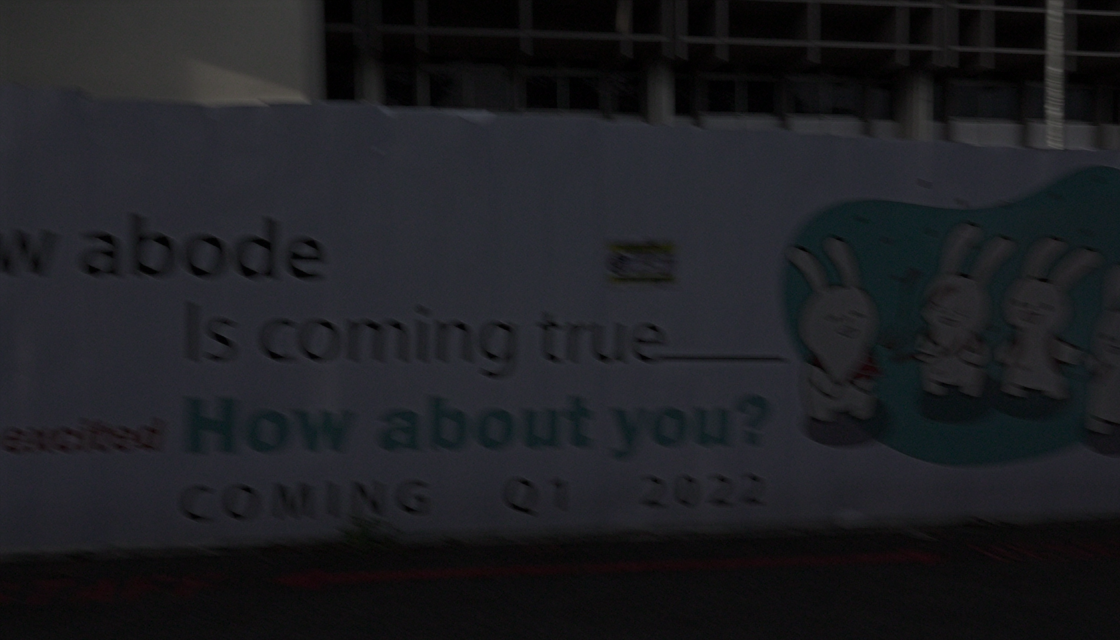
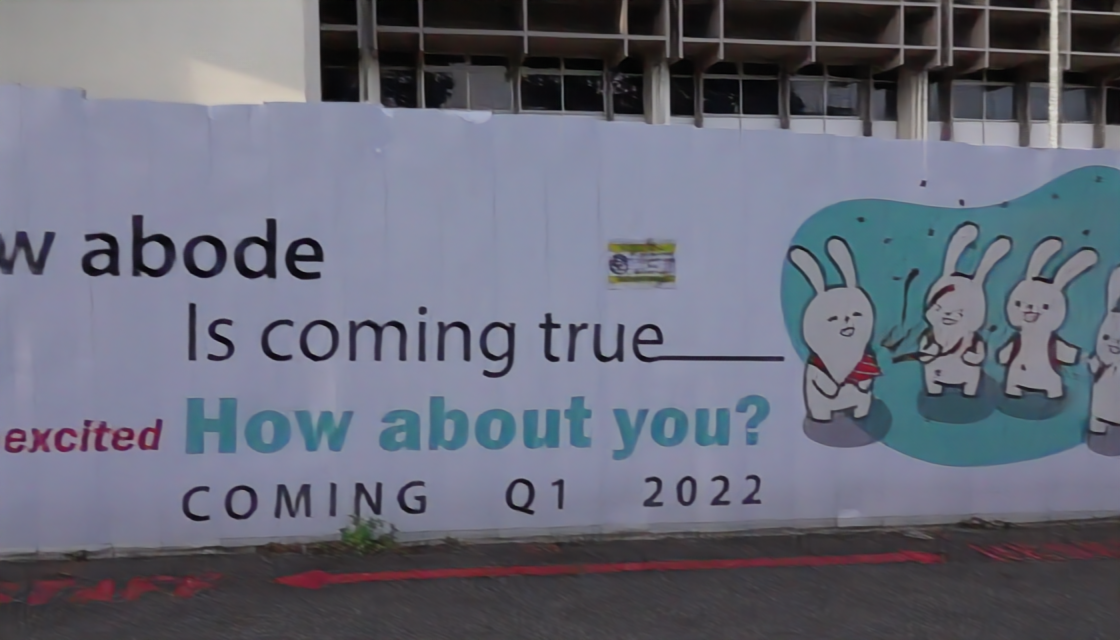