Development of an Inpainting Neural Model for Autonomous Driving
Explore the innovative methods we employ to develop a complete set of driving data and train an advanced machine learning model for Inpainting in autonomous driving.
Creating the autonomous driving dataset
Artificial generation of the dataset from BDD100K and its posterior training.
Artificial generation of images with vehicles.
Our model needs as input images, the ones that contains vehicles to remove, but as we have not found this situation in different datasets (it’s so difficult to contain exactly the same image with and without vehicle to remove) we have take the decision of generate our dataset artificially.
As a solution, we have added different vehicles extracted from the BDD100K set in an artificial way to other images again from the same set. There will also obtain the image that in our model we will use as input, which is the one with the black mask applied on top.
Below we can see an example of this modification.
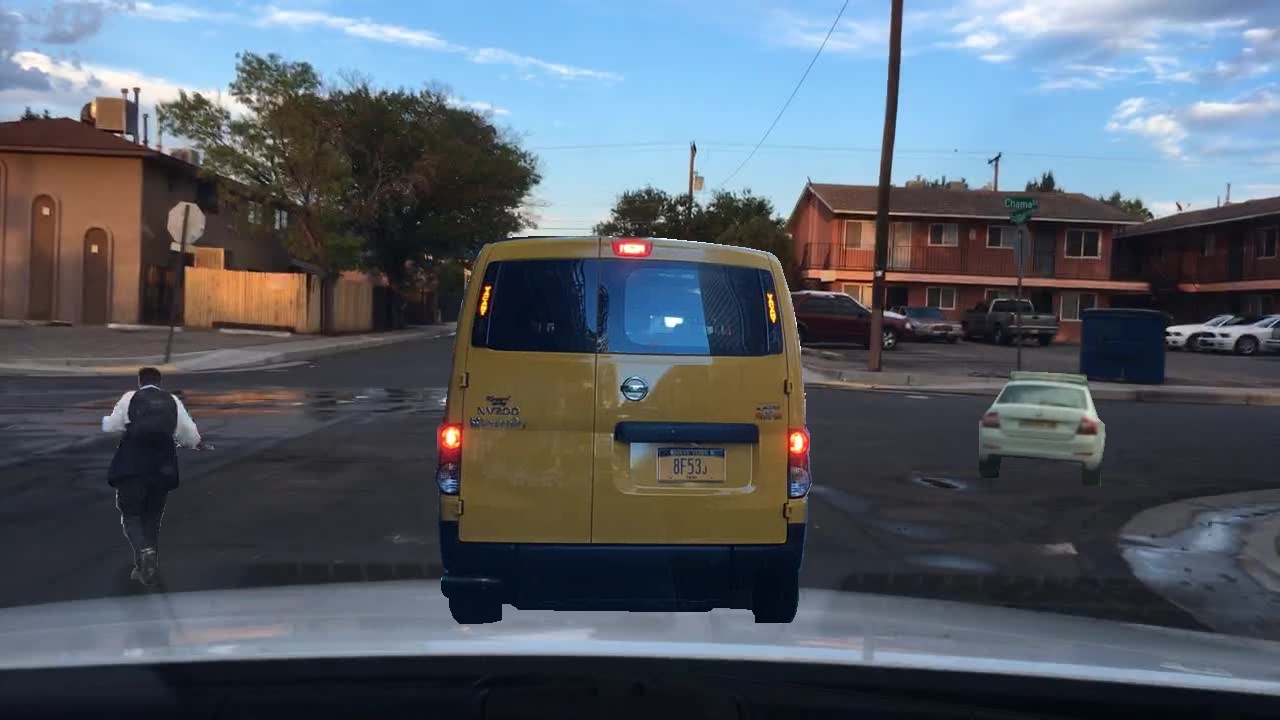
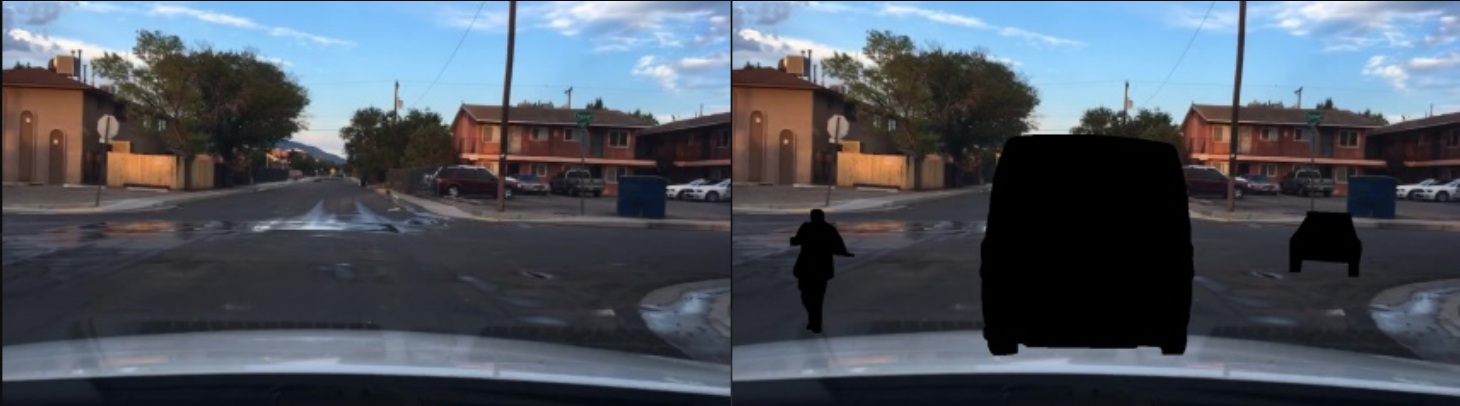
Training data preparation for our Autonomous Driving inpainting model
The training process for our neural model consists of several stages. Initially, we ensure that the input and target images consist of the structure we presented in the previous section. After that, we normalized the data and divided it into training, validation, and test sets. Finally, we simply gradually trained the neural structure we created so that it can optimally and efficiently learn from different autonomous driving scenarios.
Model RESULTS
Performance of the Inpainting Model
The machine learning model developed at the CIDAUT foundation has demonstrated remarkable accuracy in object removal. Using the BDD100K dataset as a basis, the model was able to achieve an accuracy rate of 99% in controlled tests. With this, the aim is to improve the vehicle’s ability to adapt to different driving conditions, to be able to anticipate different scenarios that have not even occurred.
Different examples of the application of the neural model on the test set are presented below.
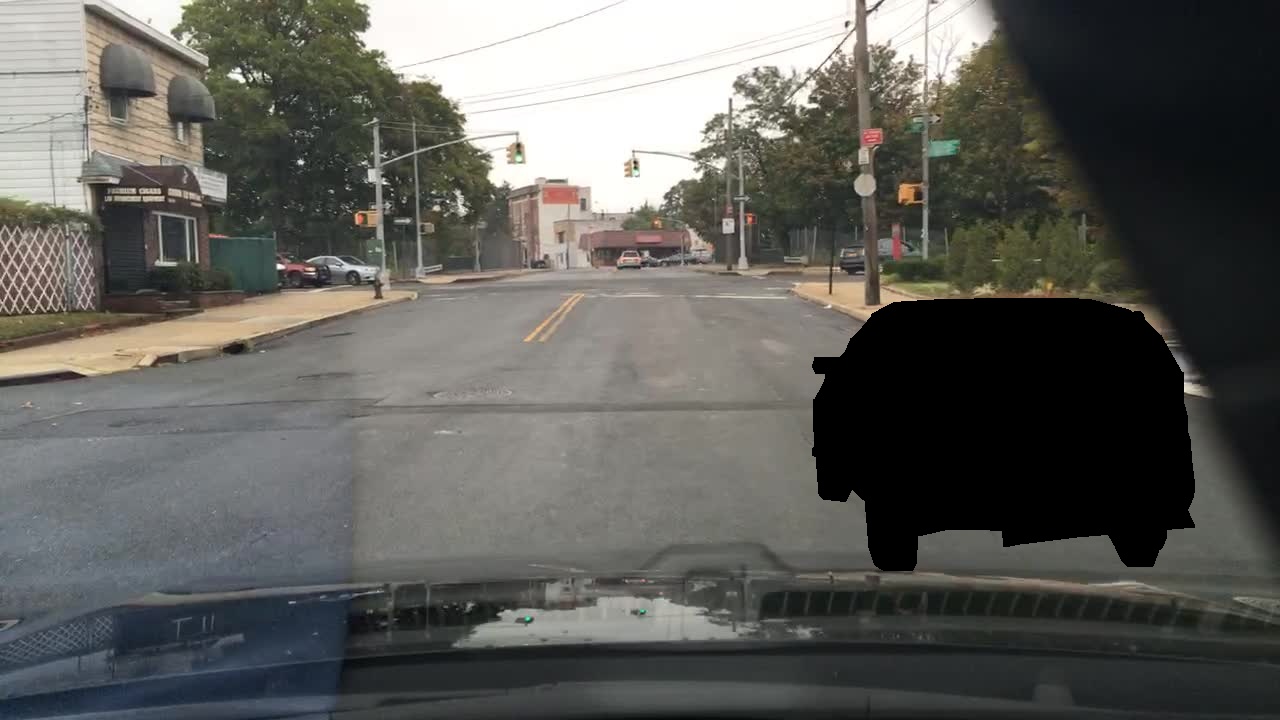
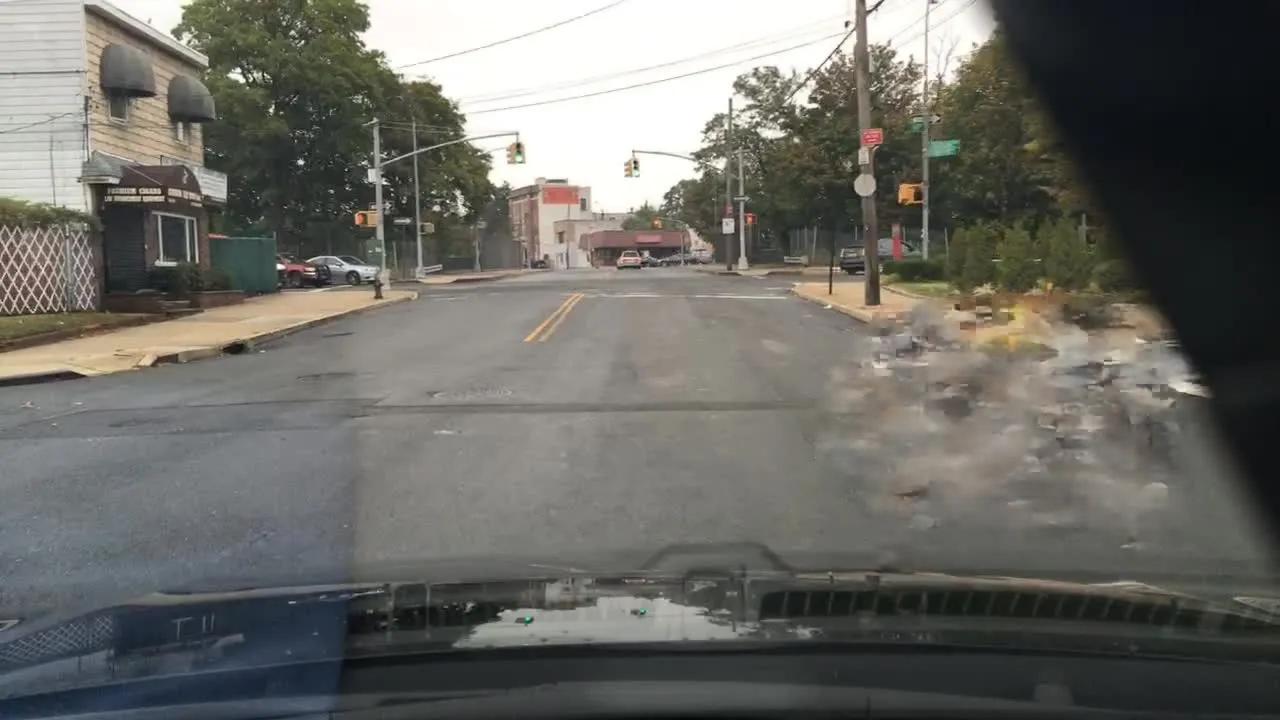
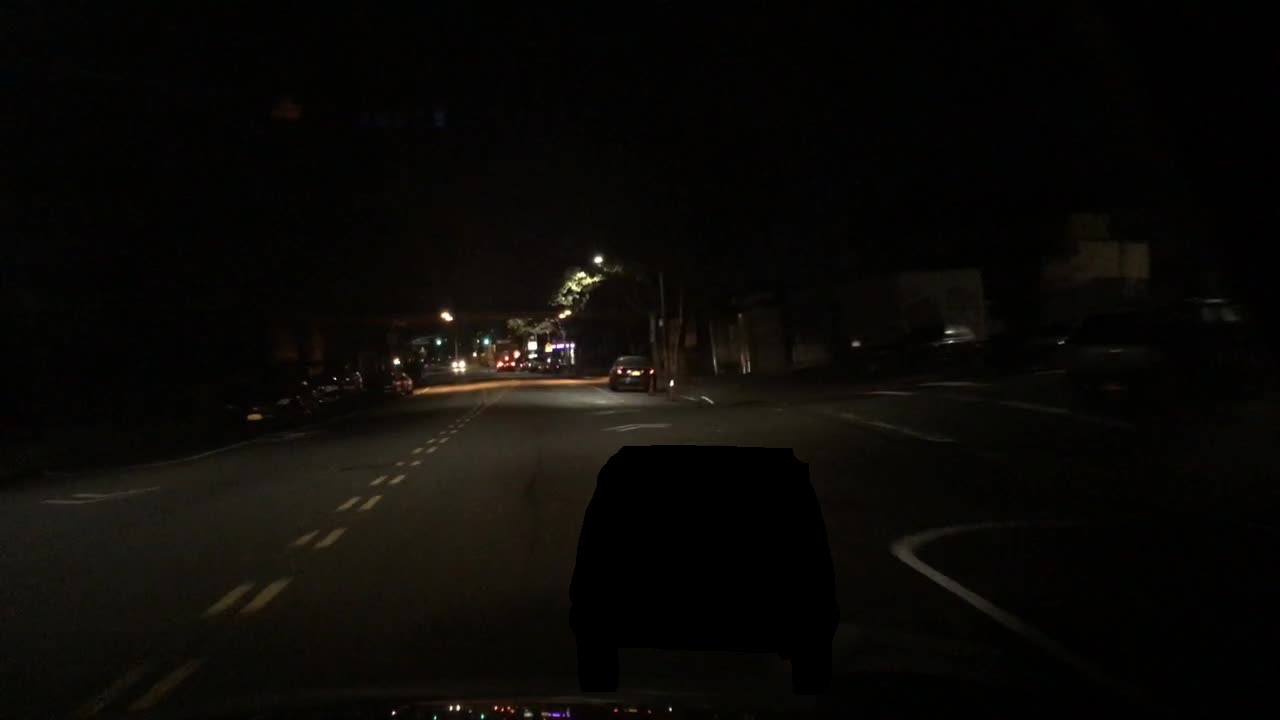
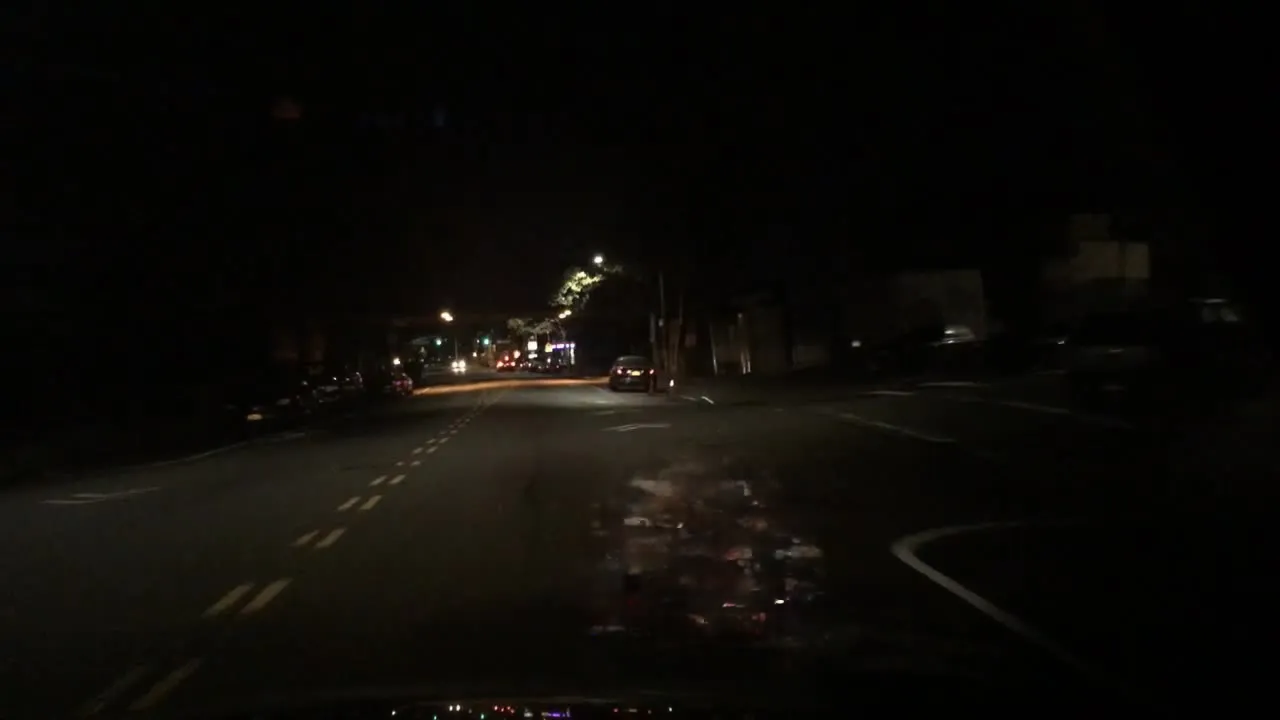